Imagine a treasure chest overflowing with unrefined gems. Extracting their true value requires careful preparation, a meticulous process known as data preprocessing. This comprehensive guide delves into the world of data preprocessing, equipping you to transform raw, unpolished data into a state that empowers machine learning algorithms to shine.
Why Preprocess Data?
Data, in its raw form, can be messy, inconsistent, and incomplete. It might contain errors, missing values, inconsistencies in format, or irrelevant information. Feeding such data directly into a machine learning model would be akin to expecting a chef to create a culinary masterpiece from unwashed ingredients and a dull knife. Here’s why data preprocessing is crucial:
Improved Model Performance: Clean and consistent data allows machine learning algorithms to focus on learning the underlying patterns and relationships within the data, ultimately leading to better model performance (accuracy, precision, recall, etc.).
Reduced Training Time: By eliminating irrelevant information and inconsistencies, data preprocessing can significantly reduce the time required to train a machine learning model.
Enhanced Algorithm Stability: Preprocessing techniques like normalization or scaling can improve the stability of machine learning algorithms, making them less susceptible to the influence of outliers or features with vastly different scales.
Facilitates Feature Engineering: Cleaned and prepared data serves as a solid foundation for feature engineering, where new features are created to capture specific relationships within the data, potentially boosting model performance further.
Data preprocessing is an essential step in the machine learning workflow. It lays the groundwork for successful model development, paving the way for accurate predictions and valuable insights.
What is Data Preprocessing?
Data Preprocessing Is the Process Of Transforms Data Into Algorithm Knowing Data. RealWord [Raw ]Data Is In incomplete and inconsistent Not Always. Make Raw-data Useful Using Data Preprocessing. Imagine a chef preparing ingredients before cooking – data preprocessing performs similar tasks on your data, ensuring it’s clean, consistent, and ready for analysis.
Data Preprocessing Step By Step
Step 1 : Import the libraries
Step 2 : Import the data set
Step 3 : Data Cleaning
Step 4 : Data Transformation
Step 4 : Data Reduction
Step 5: Feature Scaling
Import the libraries in python
First, I Import pandas and NumPy libraries and give alias.
import pandas as pd import numpy as np
Import the data set
Import Data Using Pandas. You Can Import Data: CVS, Excel, SAS, delimited, SQL And URL.
# Import CSV File Data = pd.read_csv("Train.csv") # Import CSV File Using URL Data = pd.read_csv("https://quickinsights.org/wp-content/uploads/2020/03/train.csv") # import TXT File Data = pd.read_table("train1.txt") # Import Excel File Data = pd.read_excel("train.xls",sheetname="June", skiprows=2) #Sqlite 3 db import sqlite3 from pandas.io import sql conn = sqlite3.connect('forecasting.db') query = "SELECT * FROM forecasting" results = pd.read_sql(query, con=conn) print results.head()
Data Cleaning
Find Missing Data
First, check In Data Set Have Missing Value Or Not.
import numpy as np import pandas as pd import matplotlib.pyplot as plt Data = pd.read_csv("~/Downloads/Data Science/data set/train.csv") Data.isnull()
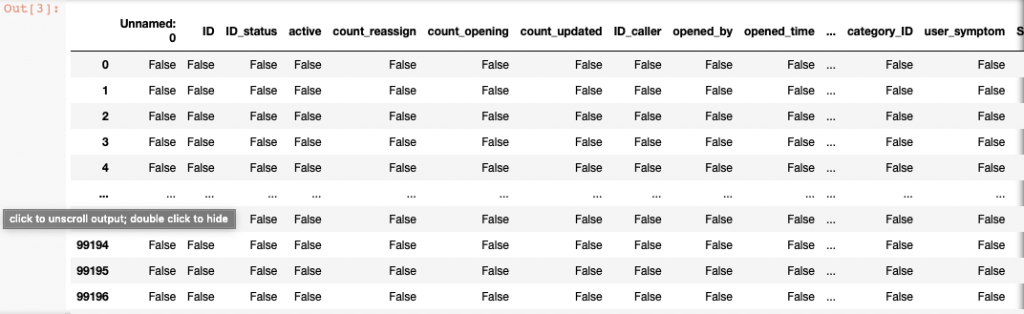
In Huge Data Set Use isnull().sum() Not Always.
Data.isnull().sum()
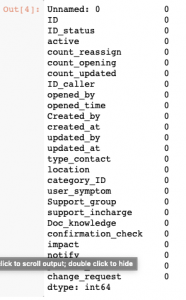
Some Time Null Value In Different Value As: ?, Blank Than Need To Convert Them NAN Format For Further Algorithm Use.
#Eliminate the NAN for col in Data.columns: Data.loc[Data[col] == '?', col] = np.nan
Visualizing Null Values
In seaborn library Use For statistical graphics visualisation
import seaborn as sns sns.heatmap(Data.isnull(), cbar=False)
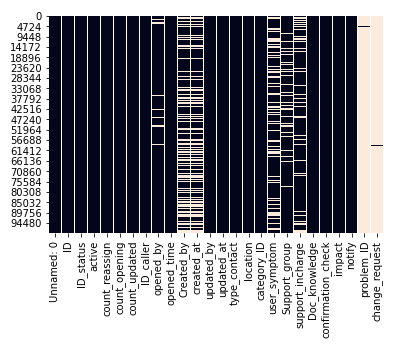
Drop Null Value
Using dropna()
Data.dropna()
Using Column name
When See Graph, and No More Use Column Or Have A 75% Null Value No fill null value For Imputation then Better Option is Remove Column.
Data.drop(['Unnamed: 0'], axis=1, inplace=True)
Filling null values using mean
# Find mean result = Data.category_ID.mode() print(result) #Then Fill null value Data.category_ID = Data.loc[Data.category_ID == '?', col] = 'Category 26' Data["category_ID"].fillna("Category", inplace = True)
fill null values with the previous and next ones
Data.fillna(method ='bfill') # for next values as Data.fillna(method ='pad') # for previous values as
fill na value using replace()
Data['category_ID'].replace(to_replace = np.nan, value = 'Category 26')
Data Transformation
When Your data is mixtures attributes Then Need Transformation them, Not Always. Example : currency, kilograms and sales volume.
Normalization
Normalization is rescaling real numeric value into the range 0 and 1.
When you don’t know data distribution or know distribution is not Gaussian distribution(bell curve). Example k-nearest neighbors and artificial neural networks.
#import sklearn library for Normalization from sklearn import preprocessing #need all value in number, not convert non number normalized_Data = preprocessing.normalize(Data)
Standardization
Standardization is shifting the distribution of every attribute with a zero mean and one standard deviation.
When your data is Gaussian distribution (bell curve). This does not require compulsory, but the technique is more effective if your attribute is Gaussian distribution and varying scale data. Example linear regression, logistic regression
standardized_Data = preprocessing.scale(Data)
Data Discretization
When Your Data is Continuous and need to convert them discrete then use Discretization.
Data['type_contact']=pd.cut(Data['type_contact'],3,labels=['email','phone','fax'])
Data Reduction
In data reduction (Dimension Reduction) techniques example: Filter method using Pearson correlation, Wrapper method using pvalue, the Embedded method using Lasso regularization. Lasso regularization is an iterative method. Each iteration extract features to check which features contribute the most to the training. If the feature is irrelevant, lasso penalizes 0(zero) and removes it. PCA(Principal Component Analysis).
#Filter method corr = Data.corr() drop_cols = [] for col in Data.columns: if sum(corr[col].map(lambda x: abs(x) > 0.1)) <= 4: drop_cols.append(col) Data.drop(drop_cols, axis=1, inplace=True) print(drop_cols) display(Data)

Feature Scaling
Feature scaling is also known as Data Transformation. It is applied to independent variables for Given data in a particular range. This is also used for algorithm speeding up the calculation.
from sklearn.preprocessing import StandardScaler scaler = StandardScaler() scaler.fit(Data)
Use Feature Scaling
Use feature scaling when data is a big scale, irrelevant or misleading, and your algorithm is Distance based. Example: K-Means, K-Nearest-Neighbours, PCA.
Common Data Preprocessing Methods
Let’s delve into some commonly used data preprocessing techniques:
- Handling Missing Values:
- Imputation: Fill in missing values with statistical methods like mean/median imputation or more sophisticated techniques like K-Nearest Neighbors (KNN) imputation.
- Deletion: Remove data points with missing values, especially if the number of missing values is high or if imputation is not feasible.
- Data Cleaning and Correction:
- Error Correction: Identify and fix typos, inconsistencies in formatting, or invalid entries within the data.
- Outlier Detection and Treatment: Identify data points that deviate significantly from the majority. These outliers can be removed, winsorized (capped to a specific value), or transformed depending on the context.
- Data Transformation:
- Scaling: Techniques like standardization (z-score normalization) or min-max scaling can ensure features are on a similar scale, preventing features with larger ranges from dominating the model.
- Encoding Categorical Variables: Convert categorical features into numerical representations suitable for machine learning algorithms. Techniques like one-hot encoding or label encoding can be used.
- Handling Skewed Distributions: Transform features with skewed distributions (e.g., applying a log transformation) to create a more normal distribution, potentially improving model performance.
Beyond these core techniques, data preprocessing might involve:
- Feature Selection: Choosing a subset of relevant features to improve model efficiency and potentially reduce overfitting.
- Normalization: Rescaling features to a specific range (e.g., 0 to 1) for specific algorithms.
Python Libraries for Data Preprocessing
Several Python libraries can streamline your data preprocessing workflow:
- pandas: Provides powerful tools for data manipulation, cleaning, and feature engineering.
- NumPy: Offers efficient array-based operations for data cleaning and transformation.
- Scikit-learn: Includes functionalities for data preprocessing tasks like scaling, encoding categorical variables, and handling missing values.
Utilizing these libraries can significantly reduce the time and effort required for data preprocessing, allowing you to focus on analysis and model building.
Conclusion
Data preprocessing, often referred to as the “unsung hero” of machine learning, plays a pivotal role in transforming raw data into a powerful tool for discovery and decision-making. By understanding the core principles, implementing common techniques, and adhering to best practices, you can unlock the true potential within your data. Remember, effective data preprocessing sets the stage for success in your machine learning journey – a journey that empowers you to extract valuable insights and drive innovation across various domains.