data science can ease the pain and increase the profit
Today’s world is a data driven world. Data science is one of the most demanding fields today. It is the extraction of the big volume of data which are structured or unstructured and used in preparation, analysis, visualization, and maintenance of information. It is a multidisciplinary field in which various technologies, scientific methods and processes are used to draw out insights from data. With the passage of time and the emergence of new technologies, data has increased across the world exponentially. In other words, we can say that data science is all about using various tools for finding raw data which is also known as mining and making changes to that data using various algorithms and accessing the refined data for the business which is known as machine learning. Data scientist helps to solve daily day to day predictions and decisions regarding business. Data scientists make fruitful decisions driven out by large volumes of data. Majority of data science consultants work in technology industry. Out of all data scientists 80% holds a master degree and 46% have PhD.
Data science and big data are both developed and designed to handle large volumes of data units. Both data science and big data have a correlated functionality but a different edge. Big data are used to store large volumes of data which is very difficult to process accurately using traditional methods on a single computer. On the other hand data science is mainly focused upon scientific approach involving scientific tools, models and techniques to process the big data. Data science supports the organisation to make the decisions.

How Applications of data science succeeds
Finance
Finance and data science always go hand in hand because finance is all about data. Data science plays a vital role in Finance industry. Financial industries are one of the earliest industries using data analytics to draw insights for better functioning of the industry. Data science providers ease the pain of these industries.
1. Data management for customers
Customer data management is a process which is used to extract data from customers and customer interactions from different source system. Huge volumes of data is mainly collected through social media and transactions. Data is mainly known to be present in two forms called as structured data and unstructured data. Structured data is quite easy to handle than unstructured data. Unstructured data is known to cause various problems which can be handled with various NoSQL tools and processed with the help of MapReduce. Various tools of artificial intelligence like Natural Language Processing (NPL), data mining and text analytics generate insights from the data about the customers and extract business intelligence. While machine learning algorithms through thorough analysis of customer data analyse the financial trend and changes in the market.
2. Detection of frauds
The danger of fraud have increased in the financial institutions. The danger of fraud is directly proportional to the number of transactions. However with the growth of data science analytics it is now possible to track down the frauds by financial institutions with the help of data scientists. One of the major fraud practised in financial industry is credit card fraud. Fraud detection accuracy have increased due to improvement in algorithm. Various types of machine learning tools are used to detect anomalies in trading data and alert the financial institutions. Other than credit card frauds there are insurance related bank related frauds which are detected by clustering algorithms which segregate the data that seems to be highly suspicious.
3. Personalized services
Financial institutions use speech recognition and natural language processing software for better interaction with their customers. The feedback is provided back by the users. Hence these financial institutions are able to take actionable insights of their customer’s need and offer better services to them.
4. Customers analysis
Data scientists are able to take insights from customer behaviour hence able to take appropriate business decisions. Various financial institutions like insurance companies make use of this strategy to increase their cross sales, measurement of customer’s lifetime value and reduce the below zero customers for optimising the losses.
5. Risk analysis
It is one of the key areas of data science. By using risk analysis and management a company is able to take various important decisions which can increase trustworthiness and security of the institution. Risk analysis is the measurement of frequency of loss and multiplication of it with the gravity of damage. Data is the important core of it. There are a large number of risks that a company can face including risks from competitors, credit and market. Various steps for managing the risk are identifying the risk and monitoring and prioritising it. Another important aspect of risk analysis is to verify the creditworthiness of customers. For this data scientist use machine learning algorithms to analyse the transactions made by the customers.
Banking
Banking – It is one of the most important application of data science. By using data science banks can manage their resources very effectively. Hence banks can make better decisions through fraud detection, management of customer data, risk analysis, customer segmentation and real time predictive analysis.
1. Lifetime value of customers
Customers play vital role in banking industries. Customer’s lifetime value is also assessed by banks which help them to monitor the customers they have and using the predictive analytics which classify and assign the potential customers to invest the company’s resources on them. Various tools such as Classification and Regression Tress (CART) and Generalised Linear Model (GLM) are used to monitor the customers of bank and also contribute towards the growth and profitability of the company also.
2. Segmenting customers
Banks classify the customers by their behaviour and common characteristics for addressing them correctly. For this purpose they use machine learning techniques like clustering and classification. One of the most popular clustering technique is K-means which is used to cluster the data on similar points.
These are some ways in which segmentation of customers is helping the banks-
- Customer identification on the basis of their profitability.
- Segmenting customers on the basis of bank service usage etc.
3. Real time analysis
Machine learning is a very important tool for the analytical strategy of various banks. With the increase in the amount of data there are various opportunities for the data scientist to make out a strategy that can be helpful for the business. Analytical techniques are mainly divided into 2 types :
Real time analytics
Allows the consumers to understand the problems that disrupt the businesses and also consider the current scenario and take decisions accordingly.
Predictive analysis
Help the consumers to follow up the right technique to solve the problem. The predict the near future problems and their suitable solutions.
4. Recommendation engines in banking
Banks also provide customised experience to their clients based on their transaction and personal information and suggest them offers and services accordingly. A data scientist take all the user data of the consumers from the previous search history, transaction history and profile data to analyse and predict the most suitable item that may interest the customers. Recommendation engines can be built either by collaborative filtering or by content based filtering algorithm. The collaborative filtering method is of two types that is customer centric or item centric. It is used to provide that the recommendations to the new users. On the other hand content based filtering algorithm recommends the most similar items to the users which they have interacted during their previous purchases.
5. Customer support
Providing customer support to the users or helping the customers to use different services help the companies to engage with their customers for long period of time. Thus helping the banks to have a better interaction with their users. There are various ways through which customer services can be provided such as replying to the customers questions, complaints and providing services according to their feedback. Data science is also helping the banks in customer support services thus providing better and accurate responses to the customers.
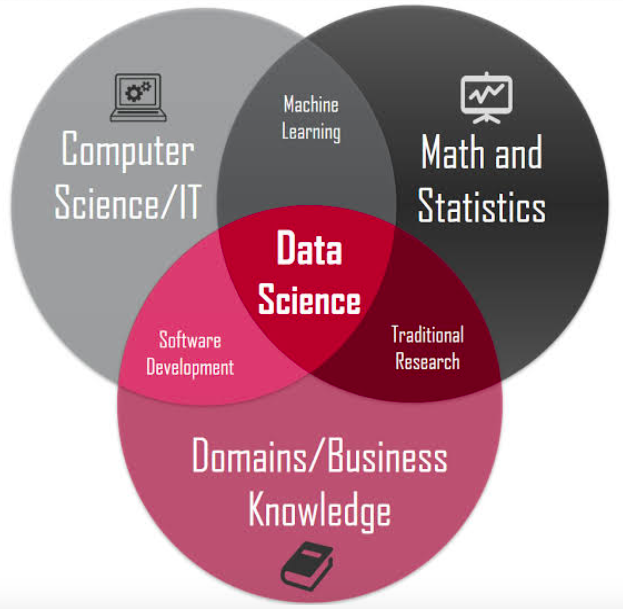
HealthCare
it is one of the most valuable application of data science. Data science has the potential to revolutionize the health care system. Medical industries are making use of data science for various purposes. At the healthcare industry is now flooded with large volumes of data that it has never seen before such as gene expression and DNA sequencing.
1. Imaging
X-rays, MRIs, CT-scans are being analysed using data science which were previously determined by doctors for other medical examiners manually. With the advancement in technologies such as computer technology and data surge. Recognition tools developed by data scientists help the medical examiners to decide the complex medical imagery.
2. Genetic studies
In genomic study data science is used to analyse the genomic sequence of various genes. Data science also helps the geneticist to understand the defects in genetic structures which help to recognise the disease and classifying it on the basis of genetic structure. We can also analyse reaction on genes due to various medicines. Sequencing time of genes have also been decreased with the help of data science. Various tools used in genomic studies are MapReduce, SQL, Galaxy and Bioconductor.
3. Discovery of drugs
Another important application of data science in the healthcare system is the discovery of various drugs. Many industries are heavily dependent on data science the formation of drugs. Drug discovery is a very complex process which require large amount of time and money and can be simplified by data science as it helps to calculate the success rate of various newly discovered drugs. This information is used by various scientist to design drugs by developing models and finding statistical relationship between various attributes. With data science, we can also analyse the reaction of various drugs on different genes with the help of machine learning technique and scientists are also able to find the probability of the development of any particular disease in the human system.
4. Prediction
A data scientist also has the ability to predict the outcome of various diseases based on the basis of historical data and pattern of disease in the patient. This ability of prediction is useful for improving the health of the patient from various chronic diseases. Population Health management is becoming popular these days which focuses on prevention of those diseases that are prevalent in the society. With this strategy hospitals can also predict the patient’s health which can become deteriorated in the near future and start an early treatment which helps in reducing the risk of the aggravation of the patient’s health.
Online business
Various industries such as e-commerce and retail industries have been hugely benefited from the data science. Data science helps to find the potential customers, forecasting of goods and services, predicting the styles and trends of products and optimising the pricing structure for the consumers. Data science is the backbone of advanced recommendation system through which insights have been provided to the customers based on their historical purchases. Hence providing better services to the customers. Data science companies create model using existing data so an organisation can learn which path will bring the best business decisions.
So we can say that data science applications are used in various different fields like finance, banking, healthcare and e-commerce. In the field of medical science data science is used for the improvement of the lifestyle of the patients and predicting diseases at the early stage of their lives. It furthermore helps in image analysis which helps to detect the microscopic tumors. In the field of banking data science is utilised for providing better services to the customers and plays an important role in various bank activities such as fraud detection, customer support services and development of recommendation engines. In the field of finance data science is used in risk analysis, customer analysis and various other places which help the organisation to increase the profit. Hence, we can conclude that data science is a vast field and its applications are also enormous and diverse and has revolutionized the world.