The Indian Premier League (IPL) tournament will be contested by 8 teams who will be playing in a “home-and-away round-robin system“, with the top four at the end of the group phase progressing to the semi-finals. in 2024 total 10 team play in IPL.
The Indian Premier League (IPL) pulsates with excitement, captivating cricket fans worldwide with its high-octane matches and nail-biting finishes. But what if you could leverage the power of technology to gain an edge in predicting the outcome of these thrilling encounters? Enter the fascinating world of Machine Learning (ML) and its potential application in IPL prediction.
Application
The main objective of this project is to predict ipl Semi-final and Final based on the future team records.
1. Data Collection
I scraped data from Wikipedia And IPLT20 Website Comprising of record of teams as of ill 2020, details of the fixtures of 2020 ipl, and details of each team’s history in the previous ipl. I stored the above piece of ipl data in three separate CSV files. For the fourth file,
I download ipl data-set for matches played between 2008 and 2019 from Kaggle in another CSV file. Then I did manual data cleaning of the CSV file as per my need to make a machine learning model out of it.
2. Data Cleaning And Formatting
Load Two CSV File. results.csv contain IPL match dates, team name, winning team name, ground city name, and winning margin. IPL 2020 Dataset.csv in appearances, won the title, play semifinal, and play final. and the current rank I give based on winning the IPL trophy.
IPL = pd.read_csv('datasets/IPL 2020 Dataset.csv') results = pd.read_csv('datasets/results.csv')
IPL.head()
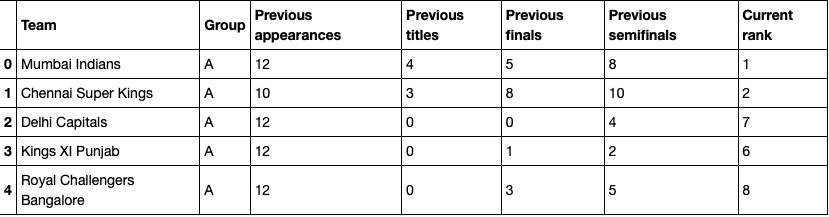
results.head()
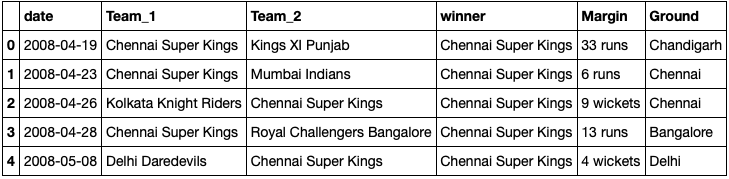
df = results[(results['Team_1'] == 'Chennai Super Kings') | (results['Team_2'] == 'Chennai Super Kings')] india = df.iloc[:] india.head()
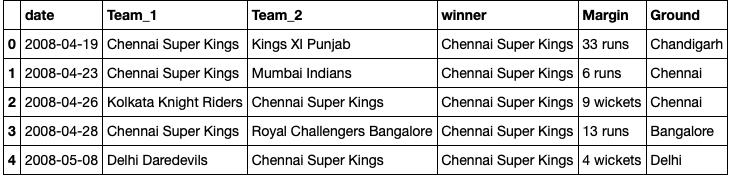
3. Exploratory data analysis [EDA]
After that, I merge the details of the teams participating this year with their past results.
IPL_Teams = ['Mumbai Indians', 'Chennai Super Kings', 'Delhi Capitals', 'Kings XI Punjab', 'Royal Challengers Bangalore', 'Kolkata Knight Riders', 'Sun Risers Hyderabad', 'Rajasthan Royals'] df_teams_1 = results[results['Team_1'].isin(IPL_Teams)] df_teams_2 = results[results['Team_2'].isin(IPL_Teams)] df_teams = pd.concat((df_teams_1, df_teams_2)) df_teams.drop_duplicates() df_teams.count()
df_teams.head()
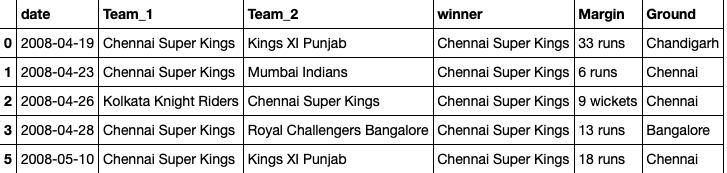
I remove the columns like date, margin, and ground. Because these features are not important for prediction.
#dropping columns that wll not affect match outcomes df_teams_2010 = df_teams.drop(['date','Margin', 'Ground'], axis=1) df_teams_2010.head()
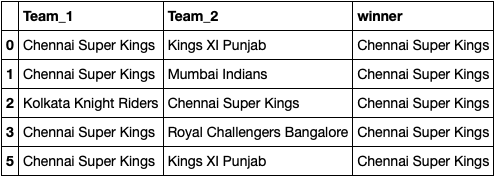
4. Feature engineering and selection
I create two labels. label 1, team_1 won the match else label 2 if the team-2 won.
df_teams_2010 = df_teams_2010.reset_index(drop=True) df_teams_2010.loc[df_teams_2010.winner == df_teams_2010.Team_1,'winning_team']=1 df_teams_2010.loc[df_teams_2010.winner == df_teams_2010.Team_2, 'winning_team']=2 df_teams_2010 = df_teams_2010.drop(['winning_team'], axis=1) df_teams_2010.head()
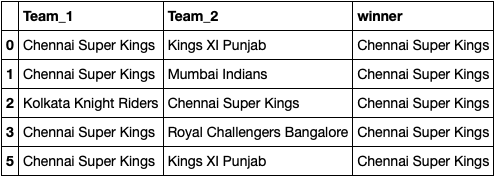
Create Dummy Variables to convert categorical to continuous
# Get dummy variables final = pd.get_dummies(df_teams_2010, prefix=['Team_1', 'Team_2'], columns=['Team_1', 'Team_2']) # Separate X and y sets X = final.drop(['winner'], axis=1) y = final["winner"] # Separate train and test sets X_train, X_test, y_train, y_test = train_test_split(X, y, test_size=0.30, random_state=42) final.head()
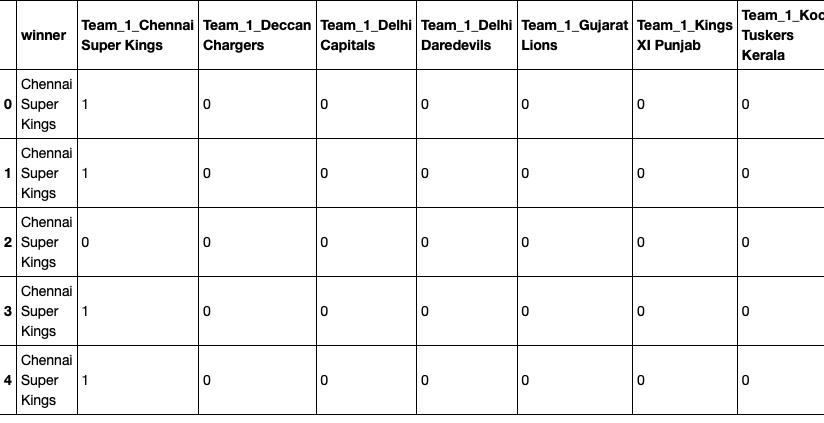
In Logistic Regression, Random Forests and K Nearest Neighbours for training the model. I chose Random Forest.
5. model building
rf = RandomForestClassifier(n_estimators=100, max_depth=20, random_state=0) rf.fit(X_train, y_train) score = rf.score(X_train, y_train) score2 = rf.score(X_test, y_test) print("Training set accuracy: ", '%.3f'%(score)) print("Test set accuracy: ", '%.3f'%(score2))

Evaluate model for the testing set
fixtures = pd.read_csv('datasets/fixtures.csv') ranking = pd.read_csv('datasets/ipl_rankings.csv') # List for storing the group stage games pred_set = []
Next, I added new columns with the ranking positions for each team and sliced the dataset for the first 56 games.
fixtures.insert(1, 'first_position', fixtures['Team_1'].map(ranking.set_index('Team')['Position'])) fixtures.insert(2, 'second_position', fixtures['Team_2'].map(ranking.set_index('Team')['Position'])) # We only need the group stage games, so we have to slice the dataset fixtures = fixtures.iloc[:56, :] fixtures.tail()

add teams for a new prediction dataset based on the rank position of each team.
for index, row in fixtures.iterrows(): if row['first_position'] < row['second_position']: pred_set.append({'Team_1': row['Team_1'], 'Team_2': row['Team_2'], 'winning_team': None}) else: pred_set.append({'Team_1': row['Team_2'], 'Team_2': row['Team_1'], 'winning_team': None}) pred_set = pd.DataFrame(pred_set) backup_pred_set = pred_set pred_set.head()
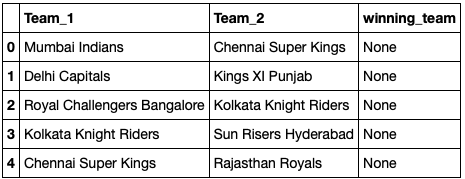
After that, Get Dummy Variables And Add Missing Columns Compare To the training model dataset.
pred_set = pd.get_dummies(pred_set, prefix=['Team_1', 'Team_2'], columns=['Team_1', 'Team_2']) missing_cols = set(final.columns) - set(pred_set.columns) for c in missing_cols: pred_set[c] = 0 pred_set = pred_set[final.columns] pred_set = pred_set.drop(['winner'], axis=1) pred_set.head()
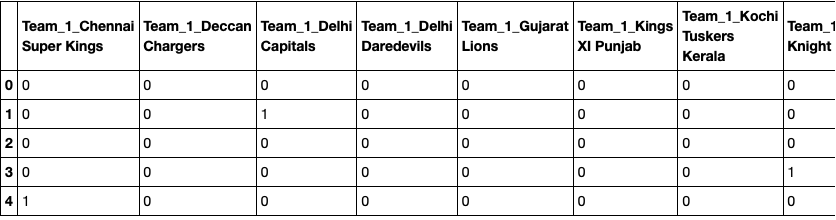
6. Model Results
predictions = rf.predict(pred_set) for i in range(fixtures.shape[0]): print(backup_pred_set.iloc[i, 1] + " and " + backup_pred_set.iloc[i, 0]) if predictions[i] == 1: print("Winner: " + backup_pred_set.iloc[i, 1]) else: print("Winner: " + backup_pred_set.iloc[i, 0]) print("")
For results, You Visit jupyter notebook Link
For Semifinal I chose Four teams Kolkata Knight Riders, Chennai Super Kings, Mumbai Indians, and Rajasthan Royals.
semi = [('Kolkata Knight Riders', 'Chennai Super Kings'), ('Mumbai Indians', 'Rajasthan Royals')]
def clean_and_predict(matches, ranking, final, logreg): positions = [] for match in matches: positions.append(ranking.loc[ranking['Team'] == match[0],'Position'].iloc[0]) positions.append(ranking.loc[ranking['Team'] == match[1],'Position'].iloc[0]) pred_set = [] i = 0 j = 0 while i < len(positions): dict1 = {} if positions[i] < positions[i + 1]: dict1.update({'Team_1': matches[j][0], 'Team_2': matches[j][1]}) else: dict1.update({'Team_1': matches[j][1], 'Team_2': matches[j][0]}) pred_set.append(dict1) i += 2 j += 1 pred_set = pd.DataFrame(pred_set) backup_pred_set = pred_set pred_set = pd.get_dummies(pred_set, prefix=['Team_1', 'Team_2'], columns=['Team_1', 'Team_2']) missing_cols2 = set(final.columns) - set(pred_set.columns) for c in missing_cols2: pred_set[c] = 0 pred_set = pred_set[final.columns] pred_set = pred_set.drop(['winner'], axis=1) predictions = logreg.predict(pred_set) for i in range(len(pred_set)): print(backup_pred_set.iloc[i, 1] + " and " + backup_pred_set.iloc[i, 0]) if predictions[i] == 1: print("Winner: " + backup_pred_set.iloc[i, 1]) else: print("Winner: " + backup_pred_set.iloc[i, 0]) print("")
then I run the semifinal function
clean_and_predict(semi, ranking, final, rf)
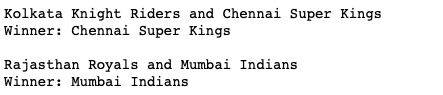
Finally, I run the final function for Chennai Super Kings and Mumbai Indians.
finals = [('Chennai Super Kings', 'Mumbai Indians')] clean_and_predict(finals, ranking, final, rf)

if this IPL 2020 final between CSK Vs MI. This Model Predicts Go To MI Side.
Full Project Code Available Click Hear
FAQ
Why i Machine Learning for IPL Prediction?
The beauty of cricket lies in its blend of strategy, athleticism, and a touch of luck. However, underlying this seemingly random sport lies a wealth of data. Machine learning algorithms can analyze this data – including player performance statistics, team history, pitch conditions, and weather patterns – to identify patterns and trends that might influence the outcome of a match.
Is Machine Learning a Surefire Way to Win?
While machine learning offers valuable insights, it’s important to remember that cricket is not a purely statistical game. Unexpected moments of brilliance, injuries, and even a dash of luck can influence the outcome. Therefore, machine learning predictions should be viewed as a supportive tool, not a guaranteed crystal ball.
How I Can Choosing the Right ML Algorithm For IPL Prediction?
There’s no single “magic” algorithm for IPL prediction. The most effective approach depends on the specific aspect you’re trying to predict. Here are some commonly used ML models:
- Logistic Regression: Powerful for binary classifications like predicting the winner of a match.
- Support Vector Machines (SVM): Efficient for handling high-dimensional data and complex relationships.
- Random Forests: Ideal for dealing with large datasets and making robust predictions.
- Neural Networks:Â Can learn intricate patterns and excel at complex predictions like the total score of a match.
In Conclusion: Embrace the Excitement, Not Just the IPL Prediction
As technology evolves, ML’s role in IPL is likely to become even more prominent. However, it’s important to remember that ML is a tool, not a crystal ball. The human element of strategy, player brilliance, and a dash of luck will always remain integral to the magic of cricket. Ultimately, ML in IPL serves to enhance the overall experience, providing valuable insights for both fans and teams, making the already enthralling sport even more data-driven and exciting to follow.