The healthcare industry stands at the precipice of a revolution, driven by the transformative power of data science. By harnessing the vast troves of medical information now available, data science is fundamentally altering how we diagnose diseases, develop treatments, and deliver patient care. This article delves into the exciting intersection of data science and healthcare, exploring its applications, ethical considerations, and the future landscape.
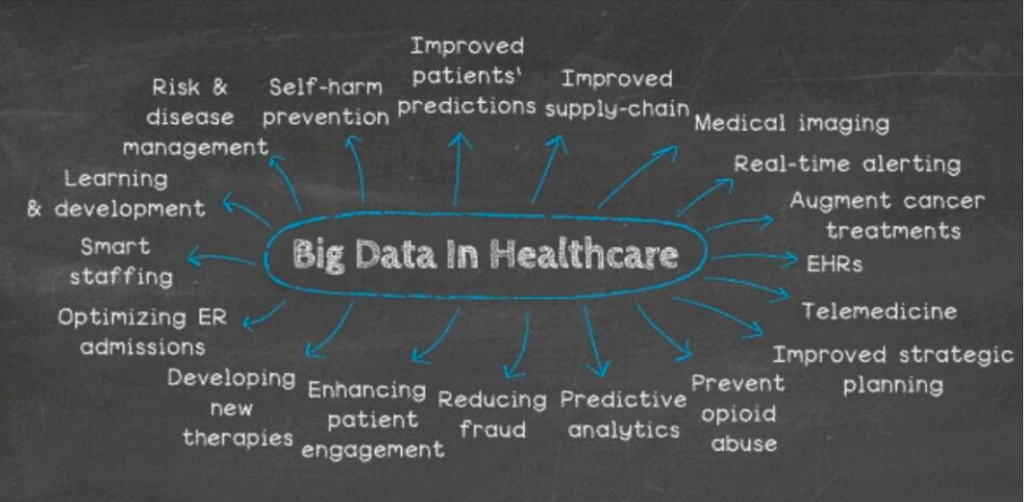
Power of Medical Data
The human body generates a symphony of data – electronic medical records, genetic information, wearable sensor readings, and medical imaging scans. Traditionally, this data has remained siloed, hindering its potential to improve healthcare. Data science acts as the conductor, orchestrating disparate data sets to extract meaningful insights. Through advanced statistical techniques, machine learning algorithms, and natural language processing, data scientists unlock the secrets hidden within this medical data.
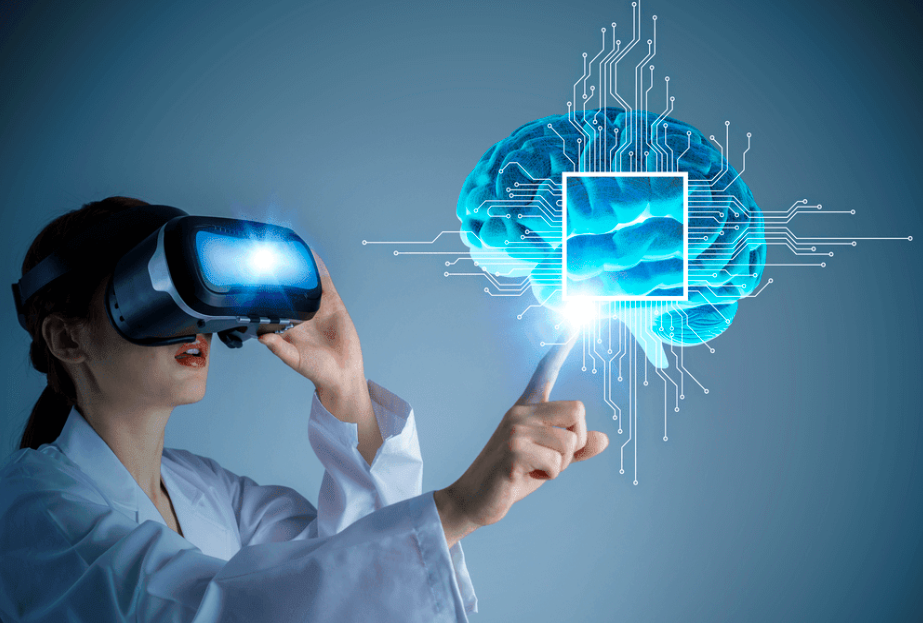
Revolutionizing Diagnostics and Treatment
One of the most impactful applications of data science in healthcare lies in its ability to improve diagnostics. By analyzing historical patient data, medical images, and genetic information, data science empowers healthcare professionals to:
- Predict Disease Onset: Algorithms can identify patterns in patient data that signal an increased risk of developing specific diseases. This allows for early intervention and preventive measures, potentially saving lives.
- Improve Diagnostic Accuracy: Machine learning algorithms trained on vast datasets can assist doctors in analyzing medical images, such as X-rays or MRIs, with improved accuracy. This can lead to earlier and more precise diagnoses.
- Personalize Treatment Plans:Â Data science facilitates the creation of personalized treatment plans by factoring in a patient’s unique medical history, genetic makeup, and lifestyle. This tailored approach can lead to better outcomes and reduced side effects.
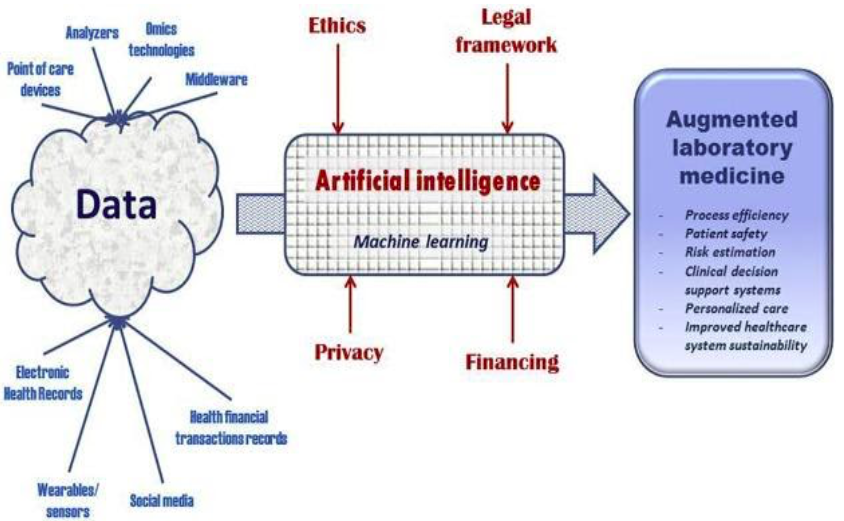
Optimizing Healthcare Delivery
Beyond diagnostics and treatment, data science is optimizing healthcare delivery systems in several ways:
- Streamlining Operations: Data analysis helps hospitals and clinics identify inefficiencies in workflows and resource allocation. This enables them to optimize scheduling, staffing, and resource utilization, leading to improved patient flow and reduced costs.
- Remote Patient Monitoring: Wearable devices and sensors that collect real-time health data empower doctors to remotely monitor patients with chronic conditions. This allows for timely intervention in case of health deterioration and facilitates proactive care management.
- Drug Discovery and Development:Â Data science accelerates drug discovery by analyzing vast datasets of biological information and chemical compounds. This can lead to the identification of promising drug targets and streamline the development process.
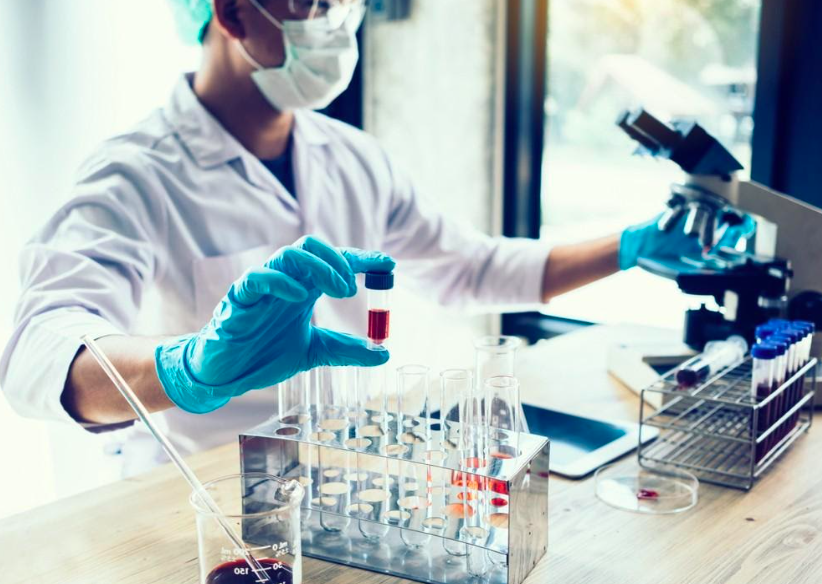
Ethical Considerations and Data Privacy
The transformative power of data science in healthcare is undeniable. However, ethical considerations and data privacy concerns require careful attention. Here are some key points to consider:
- Data Ownership and Security: Clear guidelines are needed to ensure patient data ownership and robust security measures to prevent breaches.
- Algorithmic Bias: Data science algorithms can perpetuate existing biases in healthcare if trained on biased datasets. Mitigating bias in data collection and algorithm development is crucial.
- Transparency and Explainability:Â Data-driven models used for diagnostics and treatment decisions must be transparent and interpretable by healthcare professionals. This fosters trust and allows for human oversight.
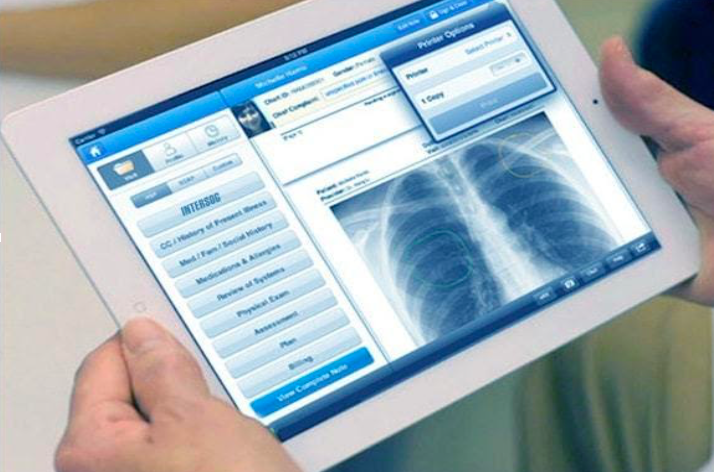
The Future of Data Science in Healthcare
As data science continues to evolve, the future of healthcare promises even more groundbreaking innovations:
- Artificial Intelligence (AI) in Medical Decision Making: AI-powered systems will play an increasingly prominent role in assisting healthcare professionals with complex diagnoses and treatment decisions.
- Precision Medicine: Advancements in genomics and data science will pave the way for precision medicine, where treatments are tailored to an individual’s unique genetic makeup.
- Personalized Health Management:Â Empowered by data-driven insights, individuals will be able to take a more active role in managing their health and preventing future health issues.
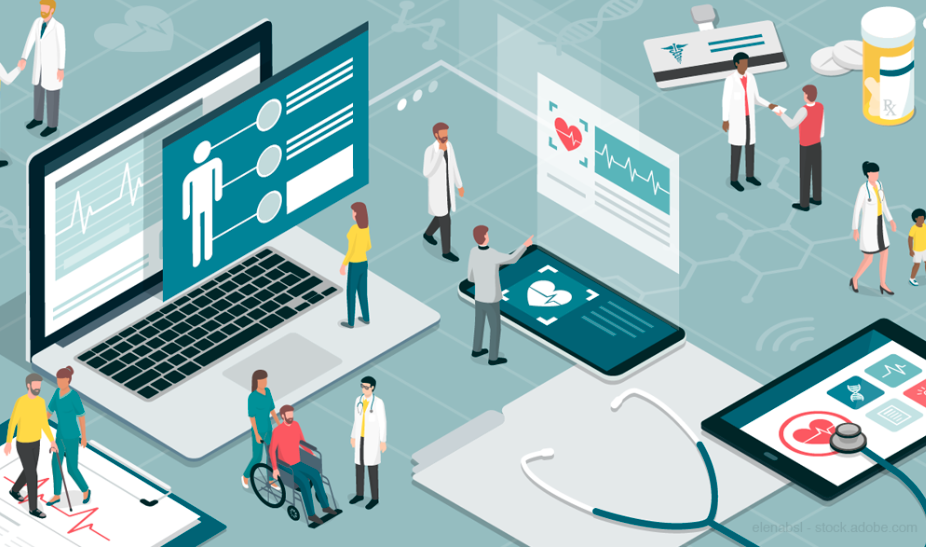
Conclusion
Data science is rapidly transforming the healthcare landscape, ushering in an era of personalized medicine, improved diagnostics, and optimized healthcare delivery. As we navigate the ethical considerations and ensure data privacy, the future of healthcare holds immense promise. By harnessing the power of data, we can create a world where healthcare is proactive, preventive, and truly personalized.